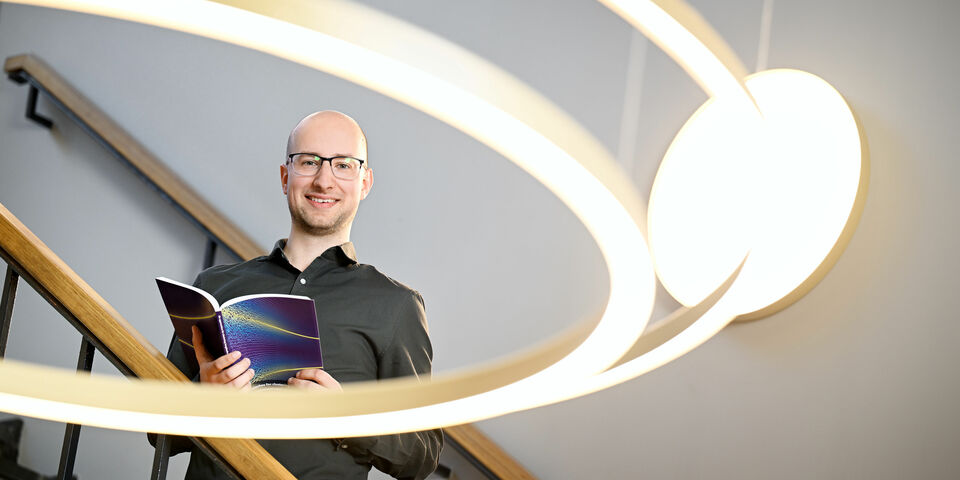
- Research , Home Stretch
- 03/07/2023
Home Stretch | Improving therapy adherence with data
The use of longitudinal data, which are based on repeated measurements at different moments in time, plays an important role in several fields, including medicine. PhD candidate Niek Den Teuling, who will be defending his dissertation at the Department of Mathematics and Computer Science on July 5, used this statistical method to map out how patients with sleep apnea use the so-called PAP therapy during the habituation period. This allows for a better understanding of the behaviors of individual patients and improved therapy adherence through early interventions.
Sleep apnea is a chronic sleep disorder that affects one in five people over 65. People with sleep apnea suffer from frequent pauses in their breathing during sleep caused by airway closure. Untreated sleep apnea has a negative effect on health; it leads to an increased risk of heart failure and strokes, among other things.
So-called Positive Air Pressure (PAP) therapy is an effective treatment method that uses a respiratory device to provide patients with increased air pressure that keeps their airways open. This requires patients to wear a mask on their face during sleep. A tube connects the mask to the device that blows ambient air into the airways.
Building a new habit
“Patients are recommended to use the therapy for a minimum of four hours for at least five nights a week, but not everyone is able to keep that up,” Den Teuling says. “Some patients may find the therapy uncomfortable. Furthermore, it is also a matter of building a new habit and that takes time for most people.” He started his doctoral research at Philips, the manufacturer of the PAP devices. The company wanted to gain more insight into how patients use the therapy during the first ninety days - the crucial habituation period in which many people either acquire this new habit, or drop it. The PhD candidate researched how often and for how many hours a day the patients would use the therapy, and whether certain trends could be discerned.
“Normally you would conduct this sort of analysis with standard statistical models, which allow you to identify the general trend of the population, i.e. the average pattern people exhibit,” he explains. “But we know that there are major differences between patients who undergo this therapy. So you could calculate the average, but that doesn’t tell us anything about the different patients. The spread in variance (the extent to which the values differ, ed.) among patients is far too wide.”
Clustering
The method he used to get more out of the data is called longitudinal data clustering. It involves looking for different patterns in the data that are similar to each other. “That way, you don't just find one general trend, but multiple trends, which also helps you better explain the individual patterns of patients.”
There are several ways to do that. In his dissertation, Den Teuling compared different models to show the advantages and disadvantages of different approaches, both within this specific domain and in a general sense. This is because there is no single ideal algorithm; the best method to use always depends on the application and the type of data you have available.
Attempts over hours
In his study, Den Teuling looked at patients who had tried the therapy for at least 90 days. His research confirmed that there were major differences in how patients use the therapy. “Just over half of the patients used the therapy fairly consistently from day one, meaning that they started wearing the mask for at least four hours a day from the beginning and actually kept it up. The other half struggled more with the therapy,” he says. In other groups, use of the therapy decreased over time, both in terms of the number of hours spent per day and the number of days the patients used it. “There were two groups that all but stopped using it altogether. So by the end of the habituation period, after about seventy days, the usage had almost dropped to zero,” according to Den Teuling.
One of his key findings was that therapy adherence is not so much determined by the number of hours the patient wears the mask, but by the number of attempts. “So above all, you have to make sure people use it on a more frequent basis to really make a habit of it, even if it’s only for three or four hours,” he points out.
Interventions
“Thanks to these models, we get a better picture of how people use the therapy and can identify groups of patients who stop using the therapy or gradually start using it less and less,” says the PhD candidate. You can perform interventions with these patients to make sure they keep up the therapy. “It has been proven that interventions, such as targeted contacting patients who are struggling with the therapy, have a positive impact on therapy adherence,” he explains. The models, which he presents in his dissertation, allow you to better identify these groups and, based on that information, intervene in a more targeted and efficient way. “You can then contact these people to help them adjust their behavior or offer them personal support, and at the same time you can stop contacting other groups that need little to no help or do that less often.”
Moreover, the models he developed can be applied in other fields as well. “Therapy adherence is an important factor in many different therapies, but the same principle applies to medication or alcohol use,” he says. In these fields, too, you can use longitudinal data clustering to discover different patterns in behavior in order to be able to anticipate them.
Den Teuling will continue working at Philips after obtaining his doctorate and will be conducting further research into therapy adherence among patients with sleep apnea. “But not necessarily using clustering; I’ll be working with other applications,” he explains. “I have also worked in other fields over the past few years, but sleep apnea has always been my focus. There is still a lot of research to be done to understand the patients' behavior and experiences even better.”
Discussion