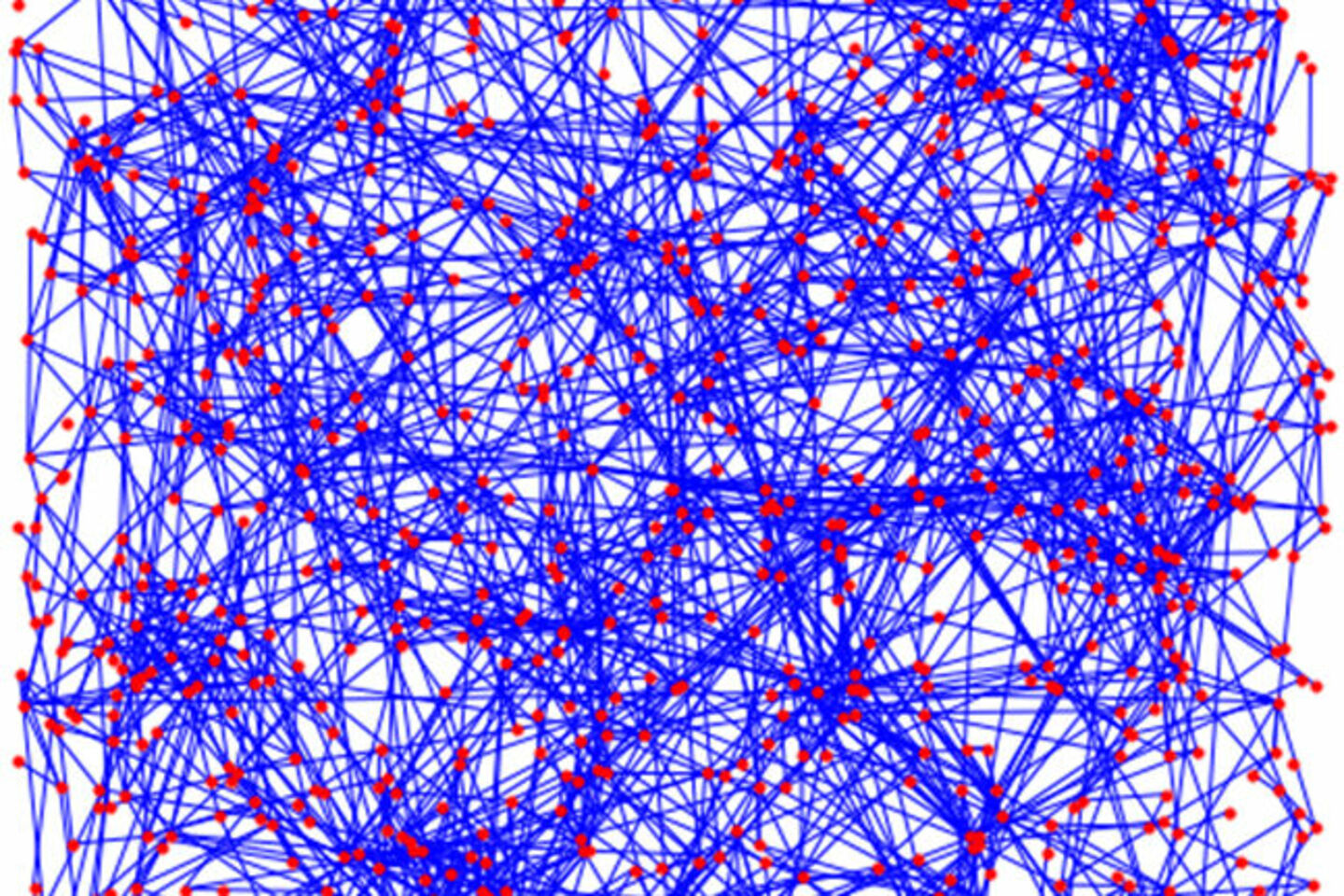
Mathematics against corona
Three PhD students working on COVID-19
Now that the first wave of coronavirus infections seems to be ebbing away, we need to arm ourselves against the anticipated second peak in the fall. That is why three PhD students at the department of Mathematics and Computer Science are working hard to analyze all facts and figures of the pandemic so far, and to predict how we can contain a new surge in infections while minimizing the impact on our economy. They recently presented their findings during the first online lunch meeting of the departmental PhD-PDEng council.
It seemed only logical that the corona pandemic was the topic of discussion at the PhD-PDEng council’s lunch meeting on Friday before Pentecost: after all, the organizers were forced to move the meeting online due to that very same pandemic. After some searching, it turned out that three PhD students from within their own ranks had decided to adjust or put on hold their regular research so that they could focus on one of today’s biggest challenges: how do we make sure that the virus causes as little damage as possible to public health and the economy in the coming period. Cursor joined the online meeting and spoke with young researchers Richard Post, Joost Jorritsma and Martijn Gösgens afterwards.
The lockdown and effective contacts
Richard Post studies for his doctorate in the group of professor Edwin van den Heuvel, who started making daily corona predictions with two of his colleagues immediately after the outbreak of the virus. Post was not that involved with these predictions, he says. “The central issue in my thesis is causality; the question of whether a correlation in data actually implies causation. That is why I focused primarily on the question of whether the government measures have had the intended effect in retrospect. We have tried to quantify that."
The most important parameter to determine this is the number of effective contact moments: how often does a person who is infected with the coronavirus come into contact with another person he or she actually infects. “In the end, that’s the number you want to reduce by implementing the measures. The number of effective contact moments is also the most important component of the reproduction number RO that health minister De Jonge has included in his dashboard, based upon the calculations by the RIVM; but for the contact moments you need less information on how long a person remains contagious; it is therefore, in my view, a better indicator of the effectiveness of corona measures.”
It seems that in Italy people started to fully comply to the lockdown only after the police began to enforce it
His model shows that the measures that were gradually implemented as of early March had an immediate effect on the spread of the virus. “We see that in other countries as well, but in some countries we see a delay. It seems that in Italy people started to fully comply to the lockdown only after the police began to enforce it."
It’s also interesting to compare the final numbers of effective contact moments in different countries after implementation of the corona measures. “You see that the numbers in Spain and Germany are lower than in the middle group, Belgium, the Netherlands and the United Kingdom, while Sweden scores highest. That’s noteworthy, because they had a complete lockdown in Spain, Belgium and the UK, and the regime in Germany was comparable to the ‘intelligent’ lockdown we had here.”
Italy | 0.29 |
Spain | 0.24 |
Germany | 0.27 |
UK | 0.37 |
The Netherlands | 0.34 |
Belgium | 0.34 |
Sweden | 0.45 |
You might draw the conclusion, Post agrees, that the intelligent lockdown has been at least strict enough, while the Swedish approach was not. But he emphasizes that the effects weren’t necessarily the result of the physical restrictions. “By closing schools, restaurants and cafes, a government gives a clear signal that the situation must be taken seriously. As a result, people immediately started to act more wisely.”
He expects to see a similar effect now that the measures are gradually being lifted. “This suggests that we’re regaining control of the situation; people will act upon that accordingly. In the end, the number of contact moments depends on the behavior of citizens.”
More details about Richard Post's research can be found in this publication.
Networks and the second wave
In the models of Post and his colleagues, it is implicitly assumed that everyone in the Netherlands has an equal chance of meeting a random fellow citizen. Although that assumption seems to work for his analysis, our society is obviously composed differently in practice – if only because inhabitants of Groningen and Limburg don’t just run into each other on a daily basis. Also, contact between people in their hometown is by no means randomly distributed: groups of friends, sports clubs and schools, among others, are at the basis of networks of people who meet each other relatively often.
Joost Jorritsma will obtain his doctorate in the group of professor Remco van der Hofstad under supervision of assistant professor Julia Komjathy for his work on the theory of these contact networks. “When the corona crisis broke out, we felt obliged to apply our knowledge in this area to this practical problem,” he says. Jorritsma believes that by presenting the population as points in a network with a geometrical component, in which people who live closer to each other are more likely to meet, you’ll get a better intuitive understanding of the measures you need to take in order to prevent a high second peak of coronavirus infections.
“For example, we have compared the effectiveness of a travel restriction to social distancing,” Jorritsma explains. “The most important thing to contain virus spread seems to be restricting contact between people who live far apart. In that case, a travel restriction would seem more effective than social distancing, should you want to choose between one of these two options.” The distance over which people would still be allowed to travel is difficult to determine, the PhD student says. “That depends on how densely populated an area is. Based on intuition I would say a few postal code areas.”
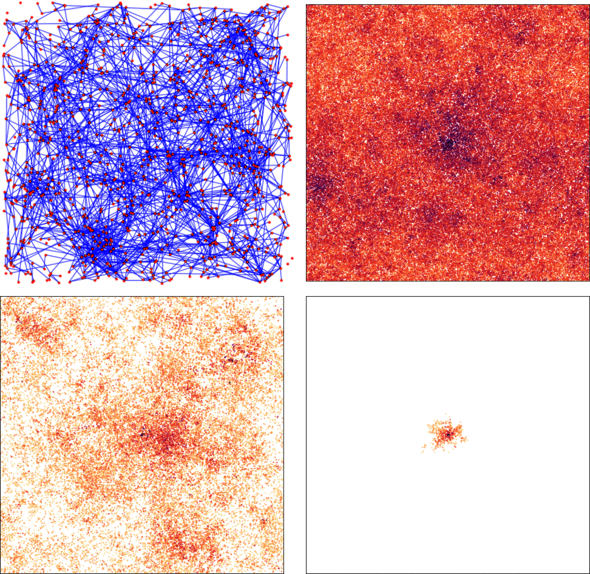
However, the results of his models are all qualitative, he emphasizes: they don’t provide hard numbers. “We haven’t been able to ‘fit’ our models to the real world so far; we are currently trying to do so in collaboration with colleagues from England. We have however entered a wide range of parameters into our models, and this leads to the same qualitative images each time.”
More about Joost Jorritsma's network approach to the spread of COVID-19 can be read here.
Economy and mobility regions
Martijn Gösgens has been working as a PhD student in Van der Hofstad’s group since a few months. He investigates the best ways to divide the Netherlands into so-called mobility regions within which people will still be allowed to travel unrestrictedly should the coronavirus flare up again. The idea is that by choosing these regions smartly, you’ll be able to find an optimal balance between containing the spread of the virus and maintaining economic activity.
The idea is to divide the country in such a way that the reproduction number stays below ‘1’ in every region, so that the number of infections won’t slip out of control. Putting municipalities under lockdown might help, but that would lead to significant practical problems. In terms of size, it’s more logical to put provinces under lockdown, but their borders don’t always correspond to the economic reality. Gösgens cites Almere as an example. The city is part of the Metropole Region Amsterdam from an economic point of view, but it’s located in a different province. “Closing the provincial borders would mean that many of Almere’s residents won’t be able to commute to Amsterdam for work. You can prevent these kinds of things by dividing the country into new regions, based on the known mobility.”
The freshly started PhD student was supposed to concern himself with the search for communities in networks, known as ‘community detection,’ Gösgens says. “Think of identifying groups of friends on Facebook, or professional fields in citation networks. My initial thought when we threw ourselves into our work on the coronavirus was that it had little ground in common with my doctoral research, but it turns out you can find mobility regions using standard algorithms from community detection.”
It seems feasible to divide the county into regions that would allow for more mobility as well as prevent infections
Gösgens used data from mobile phone networks for his models. “You can use that data to identify people’s movements between areas the size of Eindhoven’s city center.” The data isn’t more detailed due to privacy regulations. “We can’t see who has been in the same building, for example, but we don’t need that information for our research.”
Although his analysis is far from finished, it does seem that a careful choice of mobility regions can make a difference. “The exact outcomes strongly depend on a large number of corona-related parameters, such as incubation period and contagiousness, and no consensus on this has been reached yet. If we enter different parameters, the results could easily differ by one order of magnitude, but qualitatively speaking it seems feasible to divide the county into regions that, compared to Regional Health Service or provincial regions, would allow for more mobility as well as prevent infections.”
Once the results have a more solid foundation, Gösgens and his colleagues want to share these with policy makers. “Of course, we hope to make a contribution so that the entire country won’t have to be put under lockdown again. That would be great.”
Discussion