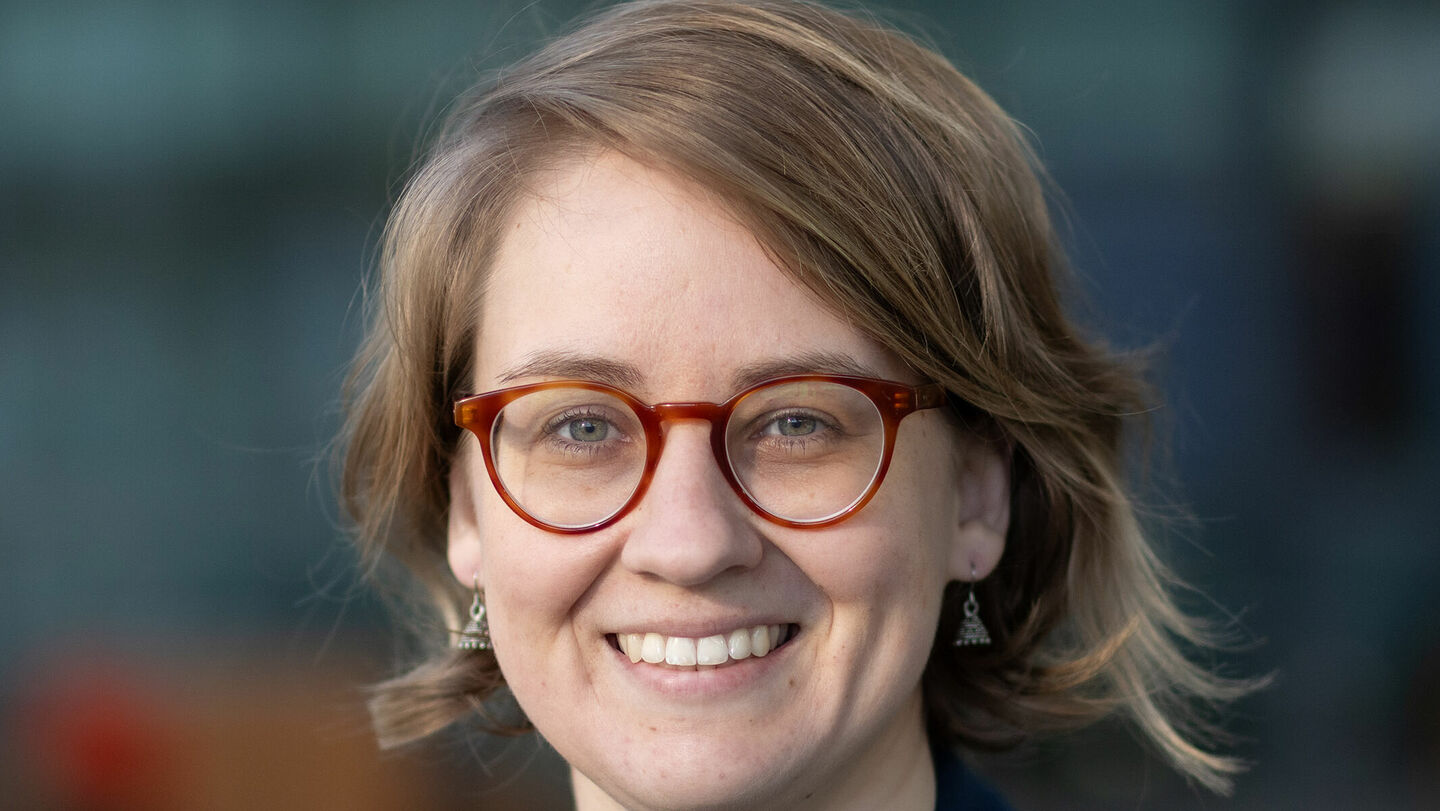
Home Stretch | Discovering unusual patterns in data
How do you discover hidden patterns in large amounts of data? TU/e researcher Rianne Schouten developed a method to make exceptional groups visible. Whether it’s young people with aberrant drinking behavior or children with learning difficulties, her work reveals surprising insights that can contribute to practical solutions in society. Today she will be defending her dissertation at the Department of Mathematics & Computer Science.
“While doing my Master’s in Methodology and Statistics at Utrecht University, I learned a lot about collecting and analyzing data,” Rianne Schouten says. “I worked mainly with data on real people and it took a lot of effort to collect them. When I applied for a PhD position in the field of Exceptional Model Mining (EMM), it was a completely new area for me, but one that immediately appealed to me.”
She explains that the idea behind EMM revolves around identifying subgroups in data sets that behave differently from the rest. “Whereas traditional statistical methods focus on analyzing the entire dataset and generating a picture of the ‘average person,’ EMM offers the opportunity to make exceptional groups visible. For example, groups of patients in whom a drug works differently, or students with an abnormal learning curve. These exceptional groups often remain invisible in traditional analyses, but with EMM you can detect them.”
Schouten emphasizes that EMM offers a different perspective than traditional statistics, which is all about general trends in the entire population. “Instead, EMM looks for patterns in small parts of a dataset,” she explains. “With this technique, you gain much more insight into the diversity within a population. Not everyone is the same, and by examining which groups of people are different, you can understand why that is. EMM revolves around two aspects: detecting exceptional behavior and describing those subgroups so that they can be clearly distinguished from the rest.”
Hierarchical data
“There were already methods for detecting anomalous patterns in datasets, but this one was particularly suitable for what is known as ‘independent data,’ so datasets where data do not influence each other,” she explains. “In practice, this is often not the case, so then you’re dealing with hierarchical data, where elements within a dataset depend on each other. Consider students who have the same teacher: that teacher’s teaching style and communication can affect student performance. In this case, data are not independent and show more similarity.”
If you don’t take these dependencies into account, you run the risk of ending up with a distorted picture and missing important patterns. Schouten therefore developed a new method to discover interesting subgroups in hierarchical datasets. She also applied this method to different datasets, which led to valuable insights.
Alcohol consumption of young people
For her dissertation, she worked with the Trimbos Institute, which annually collects data using questionnaires among Dutch adolescents. These data sets provide a rich basis for a variety of analyses. As she opens her dissertation, Schouten points to a graph charting the alcohol use of young people between the ages of 12 and 16.
“The x-axis shows different years and the y-axis shows the percentage of young people who consumed alcohol in the past four weeks,” she explained. The graph shows a steady decline in alcohol consumption, which is the result of government measures. But over time, this decline flattens out: the percentage of young people consuming alcohol stagnates.
“To understand why the trend stops, I developed a method to identify subgroups that show an aberrant behavioral pattern,” Schouten says. She points to a red line in the graph, which represents 15- and 16-year-olds who drink significantly more than average. “Although age seems like an obvious explanation, we actually want to find subgroups that are more surprising and show anomalous patterns.”
You can combine several variables – personal characteristics such as gender, type of education, or home situation – to describe unique subgroups. “For example, girls under 13 who’ve never skipped school,” she says. “Datasets contain so many subgroups that you can’t analyze all of them manually. Using mathematical methods, you can specifically look for the most interesting subgroups.”
One striking discovery was that young people in small villages show a stronger-than-average drop in alcohol consumption. “This is valuable information,” Schouten stressed. “It provides clues to explore why this group is successful in reducing alcohol use, and how those insights can be applied to other groups.”
Detecting dyscalculia
Another example comes from a collaboration with Finnish researchers, who are developing a digital learning environment for mathematical tasks in schools. “Such an environment generates an enormous amount of data,” Schouten explains. “You can see how fast students are in answering questions and whether they those answers are correct.”
Using her method, she identified subgroups of students who exhibited abnormal behavior, such as struggling with specific question types. “We found a group showing symptoms of dyscalculia,” she says. “This information can be fed back to teachers so they can provide more targeted guidance.”
A multidisciplinary approach
Schouten’s research illustrates the importance of collaboration across disciplines. “I focus on developing the method to detect exceptional patterns in data sets,” she explains. “But those patterns are often the point of departure. I pass the insights we generate on to experts such as sociologists or behavioral scientists so they can work with them.”
Especially with social issues, such as youth alcohol use or learning disabilities, an interdisciplinary approach is essential. “My work is about revealing the hidden structure in data, but the final interpretation and application of those insights is something that happens in close collaboration with other experts,” Schouten says. She hopes her research not only yields new insights, but also contributes to concrete solutions to social issues. “I think it’s very important for my work to be useful and relevant to society,” she says.
PhD in the picture
PhD in the picture
What’s on your dissertation cover?
“The little figures on the cover symbolize different people in society. By giving them different colors, you can distinguish the subgroups from each other. This clearly shows that not everyone is the same.”
You’re at a birthday party. How do you explain your research?
“I look for subgroups in society that behave differently from the rest.”
How do you blow off steam outside of your research?
“I like to read – especially novels and fantasy. It helps me to temporarily immerse myself in another world.”
What advice would you give to future PhD students?
“Invest in good communication with your supervisors from the get-go. This will prove to be immensely helpful later on. Some people want to prove themselves before they work up the courage to be more assertive, but I think you have to communicate clearly and openly from day one. The results will follow. I was lucky to have supervisors who were always open to feedback.”
What is your next step?
“I’ll stay at TU/e as a postdoc to continue my research. We want to further develop our method and eventually create a practical tool. Imagine if teachers are able to see at the touch of a button whether a student is exhibiting exceptional behavior and what the potential bottlenecks are. This would make it truly applicable, rather than just a theoretical concept in a dissertation.”
Discussion