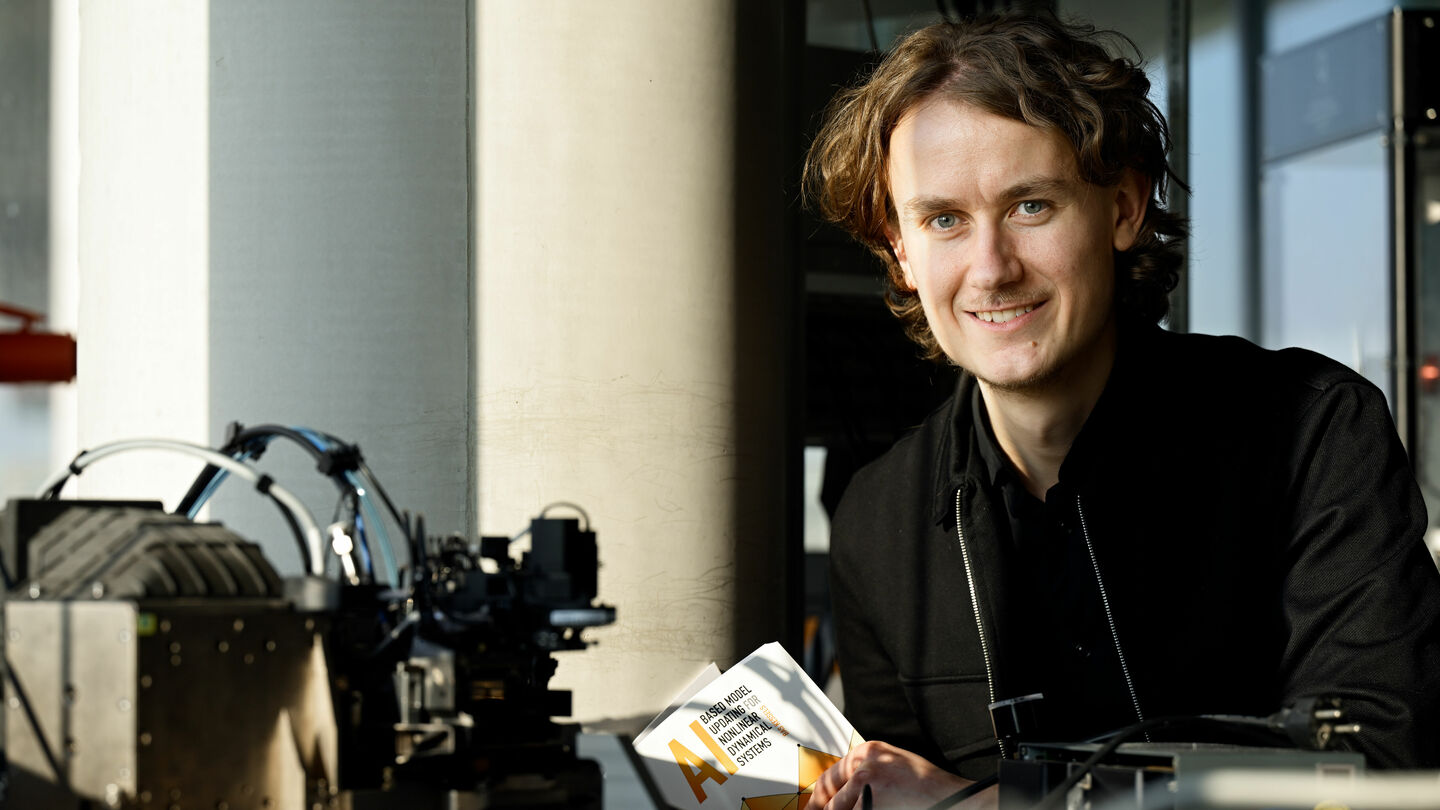
Home Stretch | Virtual copy can be increasingly accurate
Mechanical engineer Bas Kessels developed new methods for precision modelling
Earlier detection of damage to a bridge or jet fighter wing, mechanical lungs that adapt to the patient, or more efficient chip placement on a circuit board – it’s all possible thanks to precision modeling. TU/e researcher Bas Kessels developed new methods and improved models with neural networks to make digital twins of real-world systems more accurate. On Thursday, February 6, he will defend his dissertation at the Department of Mechanical Engineering.
Creating a schematic representation of reality – a model – isn’t new. Modeling has been around for years: from tangible scale models of the solar system, buildings, or bridges, to mathematical models describing all kinds of real-world systems. According to PhD candidate Bas Kessels, a good model has “infinite possibilities.” But that’s just the thing: when can you say a model is ‘good’?
“The mathematical models that are currently being made of real-world systems have physical, comprehensible laws as their basis. But we see that these laws aren’t fully capable of accurately describing all the behavior you observe in a real-world system. And so you get a mismatch between model and real-world system. Not an ideal situation if you’re using that model to make predictions or decisions for your real-world system, for example.”
Clear visualization
A new generation of computers, with ever-increasing computing power, makes it possible to use more and more measured data to map systems digitally. A ‘digital twin’ is very useful in many situations, Kessels explains. “Suppose you want to test something on a wafer scannerat ASML. It’s already difficult to be allocated time on that heavily used measurement device, and on top of that you have to be careful not to break anything on the device with your experiment. This means that it’s ideal if you have a good copy that you can subject to any experiment you like. Or take a fighter jet with a defective wing. A good digital twin recognizes the damage, after which you can run various tests to find out which maneuvers the fighter can no longer make. You then communicate this to the pilot.”
And there are many other advantages of a digital twin, Kessels says. For example, it is very efficient when you can see in the copy when damage to a piece of equipment starts to occur and then schedule preventive maintenance. In addition, you have a better view of exactly which components are defective, all of which contributes to reducing a device’s downtime. “And you can improve the performance of a piece of equipment with a good digital twin, making it safer, more accurate, and more efficient. The same is actually true for mechanics; clear visualization leads to a better workflow.”
Vibration mapping
So, a good model is comprehensible and accurate. As the latter is often lacking still, Kessels took to his computer to develop new methods that would lead to more accurate digital twins, especially of complex systems. To do so, he used neural networks, Kessels explains. “This is because you can train these neural networks based on data measured in the real-world system. And with that, the networks can compensate for the incorrect or not modeled dynamic behavior of the real-world system.” What’s unique about Kessels’ approach is that even dynamic, nonlinear systems can now be described more accurately.
Kessels then tested his new methodology at semiconductor company ASMPT in Beuningen, which wanted a more precise model of their wire bonder. This is a device used to connect chips to a circuit board, or any other electronic component. Each chip involves many gold wires that need to be connected, a lightning-fast process that takes place at the micrometer (or sub-micrometer) level. “Such a wire bonder accurately attaches wires to a chip. However, this can’t be done immediately; when the device arrives at the right location, it vibrates for a short time. Precision bonding is only possible when the device is virtually stationary. With my model, we can now map the vibration much better, so the waiting time can be shorter. And that means more chips can be handled per minute.”
Lung ventilator
The neural networks can also be used in another way, Kessels says. Here, parameter values of a model can be estimated at lightning speed based on measured data. “You can train the neural networks with simulated data. The neural network then starts to recognize patterns based on signal characteristics, to which a certain parameter value belongs. By constantly switching to the measured data from the device itself, we can adjust the digital twin with increasing accuracy.”
It’s even possible to give a degree of certainty, says Kessels, eagerly delving into a second practical example. “Something completely different – we looked at whether we could optimize mechanical lung ventilators in the hospital. Such a device helps the patient when independent breathing is no longer possible. To ventilate properly, the device must know who is at the machine: a small child, a large adult, a patient with an unknown lung problem? This requires a model that can make fairly accurate predictions in a short amount of time: what is the lung capacity, how flexible is the lung? I trained the neural network so that it can make an extremely rapid connection between the data measured in the real patient and the patient’s lung characteristics. It does so with an indication of the certainty in these characteristics, allowing a better ventilation profile to be drawn up with confidence. And if the device measures new values, it updates the model in real time, keeping it up to date.”
So thanks to these new methods, modeling can be increasingly accurate; Kessels calls it precision modeling. “To some it may seem like we’re just tinkering around, but this does constitute a next step. Not only theoretically, but also in terms of commercial application .” He smiles. “It’s never too accurate.”
PhD in the Picture
What is that on the cover of your dissertation?
“A comprehensible model, with springs and dampers. These ‘white box’ models give a mechanic lots of information. We expand that with machine learning – a black box model – and end up with a combination: the grey box. This enables us to better describe the purple signal that runs across the cover, measured on an actual wire bonder.”
You’re at a birthday party. How do you explain your research in one sentence?
“I improve mathematical models to more accurately predict the dynamic behavior of systems. Everything moves, and you want to capture that in a model.”
How do you blow off steam outside of your research?
“I make all kinds of things out of wood. From wall cabinets to a crib mobile for my little nephew. Sometimes I work with hand tools, like chisel and small saws, other times I use the table saw. Wood is very laborious, and a wonderful, living material. Those two right hands are a great combination with the theoretical thinking I do by day.”
What tip would you have liked to receive as a beginning PhD candidate?
“Know when something is good enough and don’t set the bar too high for yourself. Most PhD candidates are perfectionists to a greater or lesser degree, getting lost in details and doing way too much before they’re satisfied. But realize that you are conducting research that has never been done before and so it cannot be taken for granted that everything will immediately work as you expect it to.”
What is your next step?
“South America! I’m planning to spend about five months traveling and doing volunteer work with my girlfriend. To get a better view of the world; we basically live life in a bubble over here. If you have very different problems, your outlook in life is very different as well. We hope to experience some of this by staying in a country longer and actually participating in the community there. So my two right hands will probably come in handy again.”
Discussion