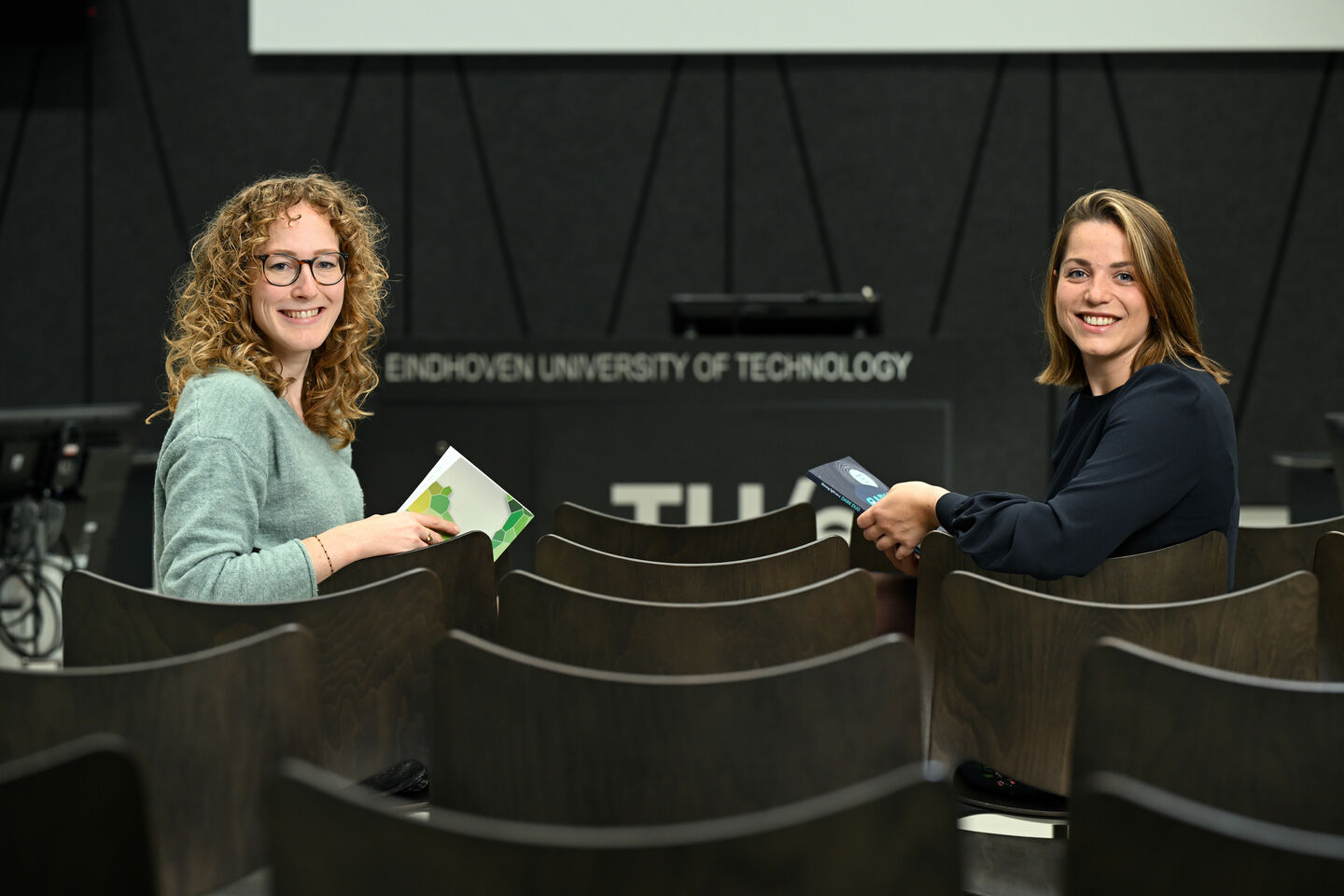
Home Stretch | Visualizing sleep
Sleep researchers Iris Huijben and Bernice Wulterkens developed new ways to measure sleep
Most people sleep between 7 and 8 hours every night. Although we are not aware of it, a lot happens in our bodies while we are lying in bed. But what if sleeping becomes a problem? To gain more insight into the sleep process, TU/e researchers Iris Huijben and Bernice Wulterkens took a closer look at current sleep measurements. They explored how machine learning helps us view sleep from a different perspective, and how this can ultimately benefit people with sleep apnea, insomnia and sleepwalkers.
As a young girl, Iris Huijben would regularly sleepwalk down the stairs, take a walk around the living room or kitchen and then be taken back to bed. The next morning, she would have no recollection of these nightly walks. However, she did often have sleepy, tired-looking eyes. Bernice Wulterkens also knows what it is like to have trouble sleeping: lying awake for hours only to drift off into a restless slumber – “It makes you jealous of people who can fall asleep instantly. That seems heavenly to me.” Four years ago, they started their doctoral research almost simultaneously in the Signal Processing Systems research group (Electrical Engineering). Two substantively distinct sleep projects, in collaboration with the Center for Sleep Medicine Kempenhaeghe. After a period full of sleep sensors, wearables, algorithms, but also drinks parties centered on sleep and even an airplane flight over TU/e, they are both defending their dissertations this week.
“When you dive into sleep research, you discover how fascinating sleep really is. It’s like a wakeful coma.” The phenomenon of sleep still amazes Iris Huijben to this day. Because we still know so little about what exactly happens when we sleep, she says. “For a long time, people thought sleep was a standby state for your body. This is not surprising, because from the outside, it looks like nothing is happening. It was only in the 1950s that our field of research began to develop, when rapid eye movements were observed during sleep. Later, it became possible to measure brain activity during the night and scientists discovered that sleep consists of multiple stages.”
Covered in sensors
So sleep is about more than just resting. During your sleep, you cycle through a repeating pattern – a sleep cycle – consisting of five sleep stages: the falling asleep stage, light sleep, the transitional stage from light to deep sleep, deep sleep and REM sleep, also known as dream sleep. Each stage has its own characteristics and functions. In a normal night, you go through an entire sleep cycle 4 to 5 times and you may briefly wake up between sleep cycles. With a polysomnography, a nighttime sleep recording, all sleep stages can be visualized, explains Bernice Wulterkens. “You’re covered in all sorts of sensors. These measure the activity of your brain, eyes and muscles, your heart rate and your breathing. We also make a video and an audio recording to link the measurements to your sleep behavior.”
“Every 30 seconds, data determines which sleep stage the sleeper is in,” Huijben adds. “So we measure a lot, but much of the data remains unused. Physiologically, a lot can happen in 30 seconds. That’s why I used machine learning algorithms to examine a great many sleep recordings. This way, we want to see if we can extract more information from the raw data, and if, outside of the sleep stages, new patterns can be discovered that we’re not able to detect with the current way of measuring. That could teach us more about healthy sleep, but also about sleep disorders, of course.”
Complex sleep disorder
Wulterkens livens up. Whereas Huijben spent a lot of time behind her computer for data analysis, she could often be found at the patient’s bedside. “There’s a group of people who are diagnosed with sleep apnea but for whom the treatment doesn’t work. In many cases, they suffer from a combination of insomnia and obstructive sleep apnea: COMISA. When a patient with suspected sleep apnea is referred to the hospital for a sleep study via their general practitioner, COMISA can easily be missed and is often only diagnosed at a later stage. And that’s worrisome, because patients with COMISA experience more symptoms and limitations than those with only one of the two conditions. In the sleep studies, we examined whether COMISA has certain characteristics that might allow for an earlier diagnosis.”
Initially, Wulterkens used data from Kempenhaeghe, which is where patients are referred to when they cannot be treated at their local hospital. These people often have a more complex sleep disorder which Kempenhaeghe is better equipped to deal with, Wulterkens emphasizes. That helped her in the search for COMISA characteristics. “Whereas sleep apnea involves many brief wakeful moments throughout the night, lasting a few tens of seconds each, we observed that in COMISA patients, these periods lasted much longer, around five minutes for example.” But when she switched to hospital data – Wulterkens also collaborated with the Amphia Hospital in Breda – she ran into practical issues. “Based on standard sleep measurements, it’s difficult to diagnose COMISA, as not every COMISA patient suffers from insomnia every night. Ideally, you’d want to monitor a patient over several nights, at home without all the wires.”
Although many advancements have been made in wireless sleep monitoring and many consumers are already tracking their sleep using wearables, Wulterkens could not use them. “Consumer devices are tested on young people with healthy sleep patterns and are not able to detect sleep disorders. Moreover, we as researchers don’t have access to the raw data, and a manufacturer can change their algorithms at any time. Not ideal for a scientific study.” Using wearables from industrial partner Philips, Wulterkens collected two weeks of (raw) sleep measurement data from people who wore this wristband at night. Then, she used a machine learning algorithm to score the sleep of patients with sleep disorders. The first clinical studies are promising, Wulterkens says enthusiastically. “With an improved way of measuring, we can better distinguish between wake and sleep. Current wearables often can’t differentiate between a patient who is asleep and a patient who is awake in bed without moving. And this is essential for a correct diagnosis.”
Sitting upright in bed
Huijben is also eager to make the switch to wearables. “We can now perform state-of-the-art machine learning with real clinical data. Models are usually fed with synthetic data sets, for example simple pictures of dogs and cats that must then be classified. With the professional data from Kempenhaeghe, we’ve managed to bring machine learning closer to the clinic.” That offers new opportunities to look at sleep patterns from a different angle, Huijben explains. “We can now represent sleep as a continuous process, just as it really is. Changes within and between sleep stages are gradual; the transitions aren’t abrupt. We can also use the developed machine learning models to examine sleep measured at home in a new way, in collaboration with industrial partner Onera Health. They are developing wireless polysomnography sensors for home measurements.”
In addition to analyzing regular sleep patterns, Huijben also used her ML model to examine data from sleepwalkers. “We still don’t understand much about why and when such sleepwalking behavior occurs; it’s a unique state of the body. This doesn’t necessarily involve physical walking, by the way. It also includes talking and suddenly sitting upright in bed. Sleepwalkers are also very difficult to wake up, and if they do wake up, they are often aggressive. With the standard way of visualizing sleep cycles, it is very hard to distinguish periods of sleepwalking in the brain measurements. Our ML models show that the longer a stretch of deep sleep, the more likely it is that sleepwalking behaviors will occur. We understand relatively little about what exactly happens during such a period. We hope this method will provide us with more insight, also through the use of Onera Health’s sensors to monitor sleepwalkers at home over several days.”
Listening to your body
Despite not having worked together directly, Huijben and Wulterkens were able to learn a lot from each other. Huijben: “Our group includes the entire sleep pipeline. I didn’t work with patients directly, but through Bernice I heard stories about the enormous impact of sleep disorders. And at the drinks parties we organized together, centered on sleep, there was room for sharing new ideas in addition to collegial conviviality.” And that also led to a completely different perspective – literally, Huijben laughs. Because both she and Wulterkens got to join their PhD supervisor Sebastiaan Overeem, who is not only a TU/e professor and somnologist but also an amateur pilot, on a flight around Eindhoven and see their work from above.
They have certainly become very aware of what bad sleep does to you, Wulterkens concludes. “What many people don’t realize is that there’s a lot they can do to improve their sleep habits. “No coffee at night, a consistent sleep rhythm, no screens. Especially the latter is a serious problem, particularly among young people who can’t put their phones down. And in today’s data-centric culture, more and more people monitor their sleep on a standard basis, but that, in turn, can be a source of sleep issues. Measure in moderation, and don’t forget to simply listen to your body.”
Bernice Wulterkens defended her dissertation “Shining light on a Dark Duo: Advancing the analysis of sleep in the assessment of COMISA” on Monday, October 14, 2024. Iris Huijben will defend her dissertation “Uncovering sleep structure through discrete representation learning” on Thursday, October 17.
PhD in the Picture – Iris Huijben
What is that on the cover of your dissertation?
“Colored shapes, representing the clusters we identified in our data using pattern recognition. On the back, there is a small black line which is part of a long EEG trace that becomes visible when you put all the dissertations in the TU/e Sleep Series side by side. Another way in which Bernice’s dissertation and mine are connected.
You’re at a birthday party. How do you explain your research in one sentence?
“Using machine learning – a branch within artificial intelligence – I’ve started a search for new ways to look at sleep.”
How do you blow off steam outside of your research?
“I enjoy being outside in nature, swimming laps in the pool or playing the clarinet. I also like going out for drinks with friends and colleagues.”
What tip would you have liked to receive as a beginning PhD candidate?
“Make sure you properly organize your data, literature and codes from the very beginning. A clear structure will save you many hours of searching at the end.”
What is your next chapter?
“I’m not making a decision about a new job yet; I’m putting that on hold for now. First, I'm going on a 4-month trip with my boyfriend, to New Zealand and Thailand. I have a penchant for hiking so I’m really looking forward to that.”
PhD in the Picture – Bernice Wulterkens
What is that on the cover of your dissertation?
“The dark duo OSA and insomnia, represented as two large letters D. The letters have faces, symbolizing two people, each suffering from one of the sleep disorders. When you tilt your head, you suddenly see a bigger face, someone suffering from a combination of the “dark duo”. He’s turning his face toward the light. Hopefully, the results of my research contribute to a faster diagnosis of this combined sleep disorder.”
You’re at a birthday party. How do you explain your research in one sentence?
She laughs: “When I start talking about my research, people often tell me about their dreams; I hear the strangest stories sometimes. Then I explain how new measurement methods allow us to look at sleep from a different perspective and that we can use this to improve diagnostics for people with sleep apnea and insomnia.”
How do you blow off steam outside of your research?
“By doing a lot of CrossFit, or just relaxing on the couch with a good book.”
What tip would you have liked to receive as a beginning PhD candidate?
“Embrace uncertainty and find joy in not knowing.”
What is your next chapter?
“I recently started working as a consultant at medical technology company Medtronic, where I also try to bridge the gap between technology and healthcare with my background in Medical Technology. How can we optimize healthcare processes, reduce waiting lists? In short: how do we make healthcare future-proof?”
Discussion