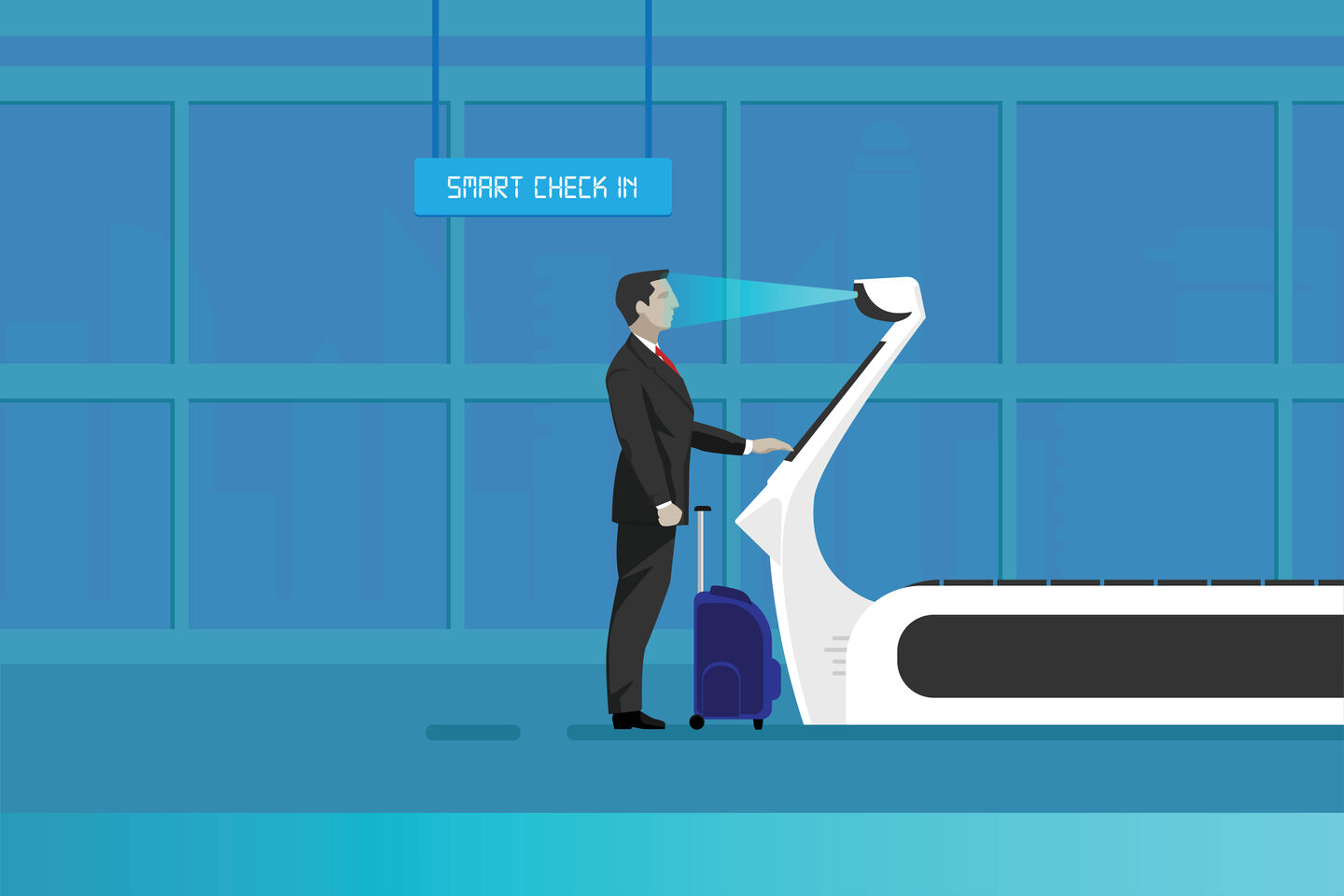
Artificial Intelligence has to be more inclusive
Three workshops are launched to help researchers make their algorithms more inclusive
The danger of self-learning algorithms (machine learning) lies in how you feed them. If the system is fed with unilateral datasets, the system evolves from there. Researcher Chiara Gallese Nobile and postdoc Philippe Verreault-Julien will host the AI & Equality workshops to help researchers to better 'educate' their systems.
Chiara Gallese Nobile is a lawyer and researcher at TU/e who is looking into AI and law. “My primary interest is how AI has an impact on individuals and society as a whole. While I was presenting results of my research in Venice, I met Sofia Kypraiou, who’s part of the organization Women at the Table that promotes equality and diversity in AI (development). They organize these AI workshops in many institutions and Sofia asked me if TU/e is also interested. I thought yes, this could fit the activities of the Ethics group at IE&IS department and the Ethical Review Board we already have. Then I contacted Philippe to help me make this happen.”
Philippe Verreault-Julien is a postdoc at TU/e and focuses his research on the opacity of AI. With a background in philosophy and ethics and an interest in AI, this topic is a match made in heaven. "Giving these workshops provides us with a great opportunity to promote a very current and important discussion. We need to combine theory and practice to apply ethical principles of the human-rights framework to systems used in society."
Problems
There is no doubt that non-diverse AI really is a problem. There are plenty of examples that proof this. Like black faces being less well recognized in facial recognition applications. “And Asians being told to open their eyes at the airport security because the AI system thinks they are not awake”, Gallese Nobile explains. “Also a famous case that could happen due to non-diverse datasets was a credit card issue. It was revealed that the card had a lower limit for females than for males and it has been accused of gender discrimination. This was due to another inequality already present in our society: the salary gap of women. The algorithm used this data to generalize to new clients.”
As far as I know there is no such thing as ethical advice before a dataset is used for an algorithm, but I would advocate that.
Gallese Nobile explains how these painful mistakes can occur: “If you put garbage in you take garbage out, meaning if you feed the machine very limited data, you can only expect so much in return. To properly feed a neural network you need lots of examples, thousands.” Verreault-Julien: “They often don’t have big enough datasets. Plus many systems are fed the same datasets. Often those sets are not diverse enough, not non-biased. They for example have more white faces than black ones, more males than females, et cetera.”
The good news: If a model is ‘ruined’ by biased data, you can re-train the model. “But that costs a lot of money and is therefore not popular” Gallese Nobile knows. “As far as I know there is no such thing as ethical advice before a dataset is used for an algorithm, but I would advocate that.”
Mostly white males
Gallese Nobile: “The workshops are aimed at helping researchers apply fundamental human rights in AI in their everyday work. The thing is, you hear a lot of talk about this topic of ethics in AI. High level discussions on when things are ethical and when not, but then nobody teaches researchers how to do it in practice. Most AI developers are white males from rich countries, often not aware of own biases.”
When asked if it’s still possible for those privileged people to represent the minorities in the datasets properly, the answer is twofold. “We need them to be able to represent themselves and not have us to speak on their behalf” Gallese Nobile says. “And even if we say we want them to be represented, there is also a political issue. We need to educate kids at a very young age, especially females. Studies found that even at 6 or 7 years of age children already think females are not very good at science, and this causes a lack of women choosing to enroll in STEM courses when they grow up. I have a son of 3 years old, and I started paying attention to the videos he watches on YouTube that are about science. It’s almost only boys there: how can a little girl feel represented if she sees that science is only for boys? It is not enough to just say we want more diversity. There is a structural inequality problem in society. It’s not just limited to the field of AI.”
Verreault-Julien is also hopeful: ”I see computer science conferences about this topic more and more. They seem really willing to apply it in AI and to learn from social scientists.”
98 percent accuracy
Gallese Nobile: “The main issue is that AI developers don’t take this gap of representation into consideration. If they don’t have the data, they just can’t make a fair system. And they don’t bother to or simply cannot collect new data and often don’t even recognize their own biases. To include more diverse samples there’s also another issue: scientists are more interested in performance than output. Let’s say they have a model with 98 percent accuracy. That will be praised in journals as it is only wrong in 2 percent of the cases. But what if that 2% is all black women from a Dutch former colony? If you use a model like that for a whole country, that 2 percent can be millions of people discriminated. So from a system perspective, 98 is very accurate, but from a human-rights perspective it is utterly wrong and discriminative.”
Taking part
The workshops are a series of three separate online sessions via Microsoft Teams. Those interested can opt for a separate workshop, or follow all three. They are free but you do need to register. Make sure you are logged on to your TU/e account as this is an intranet link.
- Thursday March 24, 'Trustworthy AI'
- March 31, 'Explainable AI'
- April 7, 'Accountable AI'
The workshops are built from a human rights principle and aim to pursue equality in AI. Learning how to feed AI systems better should ultimately lead to fairer decision making. In the workshop, research cases are discussed, as well as debiasing techniques and there is sufficient room for discussion and practice. The workshops are therefore interactive and input is expected from the participants. Gallese Nobile stresses that the workshops are aimed at researchers and could also benefit those working with AI a lot already. “The workshop is open to all those interested in the topic, but it would be helpful to already have sufficient background in AI as we will also be practicing.”
Discussion